The AI Recursion: When Machines Start Teaching Machines, and What It Means for Us
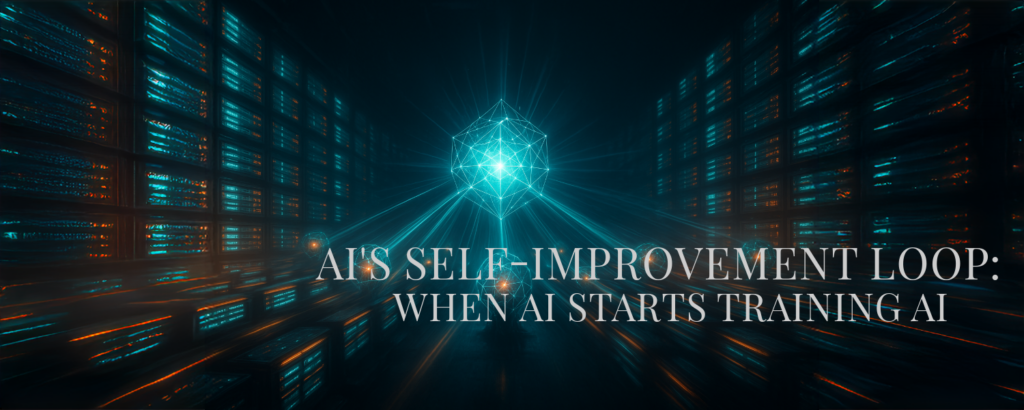
Table of Contents
Imagine a brilliant student who, after mastering a subject, becomes an even more effective teacher than their own mentor. Now, imagine this student can work 24/7, absorb information at lightning speed, and teach countless others simultaneously. This is, in essence, the electrifying concept of AI training AI. It’s a development that’s not just an incremental step, but a potential paradigm shift, fundamentally changing how artificial intelligence evolves, how fast it learns, and its eventual impact on our world.
For a layperson, understanding AI training can seem daunting. Traditionally, creating a smart AI (like the one that powers your voice assistant or recommends movies) involves feeding it massive amounts of data meticulously labeled by humans. Think of it like teaching a child to recognize a cat by showing them thousands of pictures labeled “cat.” This is time-consuming, expensive, and relies on human expertise and potential biases.
So, What Changes When AI Trains AI?
“AI training AI” means we’re introducing AI systems into this teaching role. Instead of humans doing all the heavy lifting, AIs are increasingly:
- Generating Synthetic Data: An AI can create new, artificial data (images, text, code) that looks like real-world data. This “synthetic data” can then be used to train another AI, vastly expanding the available training material without needing humans to create or find it all. Imagine an AI artist creating millions of unique cat images to train an image recognition AI.
- Acting as Evaluators and Teachers: This is a powerful concept known as Reinforcement Learning from AI Feedback (RLAIF), a sibling to the more human-dependent RLHF (Reinforcement Learning from Human Feedback). Here, one AI (the “student”) performs a task, and another, more advanced AI (the “teacher” or “evaluator”), assesses the student’s performance and provides feedback. The student AI then learns to improve based on this AI-generated critique. It’s like an AI editor refining an AI writer’s work, or an AI coach fine-tuning an AI gamer’s strategy.
- Automating Model Refinement: AIs can be designed to experiment with different architectures and parameters for other AI models, essentially trying to build better “brains” for their AI “students” automatically.
Why is This a Game-Changer? The Implications for Future AI:
The implications of AIs teaching AIs are profound, especially concerning the speed and scale of development:
- Exponential Acceleration: Human learning is linear; AI learning, especially when AI trains AI, can become exponential. AIs can work tirelessly, iterating and improving at speeds humans can’t match. This means the time it takes to develop highly capable AI models could shrink dramatically. What once took years might take months, or even weeks.
- Breaking Human Bottlenecks: Human data labelers and expert trainers are a finite resource. AI teachers can scale almost infinitely, allowing for the training of much larger and more complex models than previously feasible.
- Cost Reduction: While initial development is costly, using AI to train AI can significantly reduce the ongoing expenses associated with human labor in data labeling and model supervision.
- Novel Capabilities & Unforeseen Discoveries: AIs might develop teaching methods or identify patterns that humans wouldn’t conceive of. This could lead to AI models with entirely new, emergent capabilities that surprise even their creators.
- Hyper-Specialization: AI teachers could be tailored to train highly specialized student AIs for niche tasks with greater efficiency than a general human approach.
Impact on Industry:
The ripple effects across industries will be transformative:
- Rapid Innovation: Sectors like drug discovery, materials science, and climate modeling, which rely on sifting through vast datasets and complex simulations, could see breakthroughs at an unprecedented rate. AI-trained AIs could design new molecules, predict material properties, or create more accurate climate forecasts far faster.
- Hyper-Personalization on Steroids: From education tailoring learning paths for individual students, to healthcare creating truly personalized treatment plans, AI-trained AIs will enable a level of customization previously unimaginable.
- Automation Advancements: Manufacturing, logistics, and customer service will see even more sophisticated automation as AIs become better at learning and adapting to complex, real-world scenarios.
- New Business Models: Companies will emerge that specialize in creating “teacher AIs” or offering “AI-accelerated training services.”
Impact on the Workforce:
This is where both excitement and anxiety meet:
- Job Displacement: Roles focused on repetitive data labeling or basic AI supervision may decline as AI takes over these tasks.
- Job Creation & Evolution: New roles will emerge: AI “curators” who oversee AI teachers, ethicists specializing in AI-AI interactions, and experts in managing complex AI ecosystems. Many existing jobs will also evolve, requiring humans to work alongside highly capable AIs.
- The Skills Shift: The value of uniquely human skills like critical thinking, creativity, emotional intelligence, and complex problem-solving will increase. Lifelong learning and adaptability will become even more crucial.
Impact on Everyday Life:
The way we live, work, and play will subtly and overtly change:
- Smarter Everything: Expect more intuitive and capable personal assistants, more seamless smart home devices, and more sophisticated AI in applications ranging from entertainment (e.g., AI-generated interactive stories) to personal finance.
- Personalized Education and Healthcare: Imagine an AI tutor that perfectly adapts to your learning style or a health companion that proactively guides your wellness based on real-time data, both constantly refined by other AIs.
- Creative Tools: Artists, musicians, and writers will have access to AI collaborators that can generate ideas, drafts, or variations, pushing creative boundaries.
- The Unforeseen: Just as the internet brought changes no one predicted, the acceleration of AI development through self-training will undoubtedly lead to applications and societal shifts we can’t yet envision.
Challenges and a Word of Caution:
While the potential is immense, there are significant hurdles:
- Bias Amplification: If an AI teacher has biases (learned from its own initial human-provided data), it could pass these biases on, or even amplify them, in the AIs it trains. Ensuring fairness and rooting out bias becomes even more complex.
- Loss of Control & Understanding (The “Black Box”): As AIs train AIs, their internal workings and decision-making processes can become even more opaque to human understanding, making it harder to debug or trust them.
- Alignment Problem: Ensuring that these rapidly self-improving AIs remain aligned with human values and intentions is a critical and ongoing research challenge. We need to be sure the “student” AI is learning what we want it to learn, not just what the “teacher” AI thinks is optimal based on potentially flawed metrics.
- Security Risks: Malicious actors could try to create “evil” AI teachers to train harmful AIs.
The Path Forward:
AI training AI is not a futuristic fantasy; it’s happening now and accelerating. It promises a future where AI capabilities grow at an astounding rate, unlocking solutions to some of humanity’s biggest challenges. However, it also demands careful consideration of ethical implications, robust safety protocols, and a societal commitment to ensuring these powerful tools are developed and deployed responsibly.
The AI recursion is underway. The key will be for humanity to remain the ultimate architect and guide, ensuring that as machines learn to teach machines, they do so for the betterment of all.
Read More: AI: A Powerful Tool, Not A Thinking Mind, AIs Building Blocks: Tools, Agents, and Their Common Language (MCP) – Explained Simply
Responses